
DATA = a collection of facts from which conclusions may be drawn
MINING = the act of extracting
Now, when we combine these two words we form "DATA MINING" (Note: which also happened to be a one of our subjects under Mr. Mon Duremdes). Based on the definition given above, Data Mining simply means "extracting facts/data to be able to form conclusions". But a more profound meaning can be seen in an article of Mr. Kurt Thearling.
In his article entitled "Discovering hidden value in your data warehouse", he defined Data Mining as "the extraction of hidden predictive information from large databases and is a powerful new technology with great potential to help companies focus on the most important information in their data warehouses."

Tools in Data Mining can help businesses predict future trends and behavior "allowing businesses to make proactive, knowledge-driven decisions". This being said, we can now conclude that Data Mining, indeed, adds value to information that your company has gathered.
These Data Mining techniques are divided into two sectors namely:
-
Classical Techniques: Statistics, Neighborhoods and Clustering
-
Next Generation Techniques: Trees, Networks and Rules
Basically, these are just processes that can help you find the essential information you need in your pool of data. It's like putting all those data to undergo a "purification process"; taking value while cutting/dropping all the other cr**.
And dropping all those cr** is a very tricky thing to do. Everybody's scared of being the one to decide who goes in and who goes out. 'Cause, you see, if you let the right one out and let the wrong one in, it may be the reason for your downfall.
Data Mining is the solution to that. Instead of manually managing and analyzing those files like this: I'd rather go


I mean I'd rather be like this:
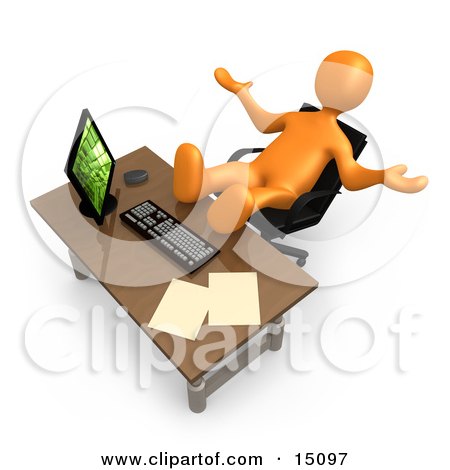
Since I happen to know this and will be able to use this process:

(Now, I will suddenly change my topic.)
Of course all these readings that my group (me, Deedin, Chabby, Ikah, and Julie; by the way, you can also check out their blogs since we pretty much talked about the same topic.) has been doing has something to do with our chosen field of study for our Data Mining class, Telecommunications.
Our professor, I think, wants us to use Data Mining to be able to find information that can be used by Telecommunications companies (especially SMART Telecom) which I'd gladly use once my group and I find all necessary information (and once we fully understand the subject and the process. LOL.)
Back to the matter at hand (which is the importance of Data Mining, I think... LOL.), here are some examples of the industries that had luck with Data Mining (taken from Mr. Thearling's Data Mining web page):
- A pharmaceutical company can analyze its recent sales force activity and their results to improve targeting of high-value physicians and determine which marketing activities will have the greatest impact in the next few months. The data needs to include competitor market activity as well as information about the local health care systems. The results can be distributed to the sales force via a wide-area network that enables the representatives to review the recommendations from the perspective of the key attributes in the decision process. The ongoing, dynamic analysis of the data warehouse allows best practices from throughout the organization to be applied in specific sales situations.
- A credit card company can leverage its vast warehouse of customer transaction data to identify customers most likely to be interested in a new credit product. Using a small test mailing, the attributes of customers with an affinity for the product can be identified. Recent projects have indicated more than a 20-fold decrease in costs for targeted mailing campaigns over conventional approaches.
- A diversified transportation company with a large direct sales force can apply data mining to identify the best prospects for its services. Using data mining to analyze its own customer experience, this company can build a unique segmentation identifying the attributes of high-value prospects. Applying this segmentation to a general business database such as those provided by Dun & Bradstreet can yield a prioritized list of prospects by region.
- A large consumer package goods company can apply data mining to improve its sales process to retailers. Data from consumer panels, shipments, and competitor activity can be applied to understand the reasons for brand and store switching. Through this analysis, the manufacturer can select promotional strategies that best reach their target customer segments.
Each of these examples have a clear common ground. They leverage the knowledge about customers implicit in a data warehouse to reduce costs and improve the value of customer relationships. These organizations can now focus their efforts on the most important (profitable) customers and prospects, and design targeted marketing strategies to best reach them.
I know what you're about to say.... "pretty cool, huh?"... Yup, I totally agree. And with that being said, I, therefore, finally conclude my blog and wish my readers a good/fun day! See yah at school!
No comments:
Post a Comment